The AI Time Horizon Test: Are We Finally Measuring Real Capability?
Written by Sabrina LowellDate Mar 20, 2025
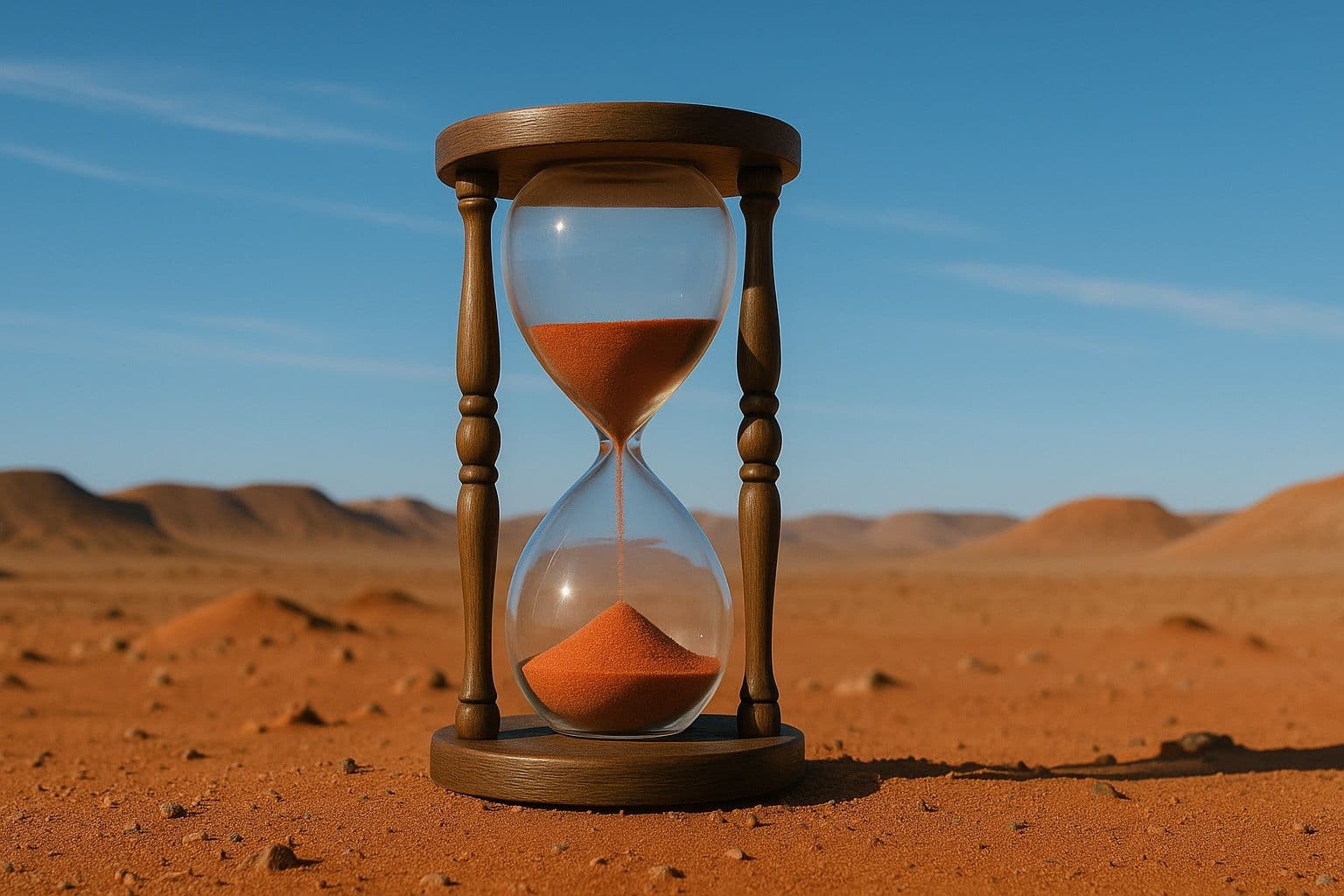
A new benchmark from METR tracks how long AI systems can reliably work—revealing steady, exponential gains in real-world task performance.
As AI capabilities continue to accelerate, so does the challenge of measuring meaningful progress. Traditional benchmarks—ranging from standardized test-style evaluations to isolated coding challenges—have offered snapshots of performance, but they often fall short of capturing how AI might perform in real-world, economically valuable contexts.
A new report from the nonprofit research group METR (Model Evaluation & Threat Research) aims to address this gap with a novel and intuitive approach: measuring how long of a task an AI system can complete with 50% reliability, compared to a human professional. They call this the “50% task completion time horizon.”
Their March 2025 paper, Measuring AI Ability to Complete Long Tasks, presents the first comprehensive attempt to quantify this idea—and the results suggest a surprisingly rapid trend.
What Is a “Time Horizon,” and Why Does It Matter?
The concept of a “time horizon” is straightforward: it reflects the duration of a task, in human professional time, that an AI model can complete with a given probability of success. METR focuses on the 50% threshold as a meaningful midpoint—high enough to imply competence, but not so strict as to require perfect reliability.
To generate this data, METR assembled a suite of 170 software and research-focused tasks, spanning everything from simple file classification (under 10 seconds) to hours-long machine learning projects. They also measured how long experienced human professionals took to complete each task, creating a baseline to evaluate AI performance in practical, grounded terms.
Then they tested 13 leading models—ranging from GPT-2 to Claude 3.7 Sonnet—using structured “agent” scaffolds that allowed the AIs to use tools like Python and Bash to complete tasks autonomously.
Their headline result: today’s top models can reliably complete tasks that take a skilled human around 50–60 minutes. And that number has been doubling approximately every seven months since 2019.
A New Lens on Progress
This exponential trendline is striking not just for its speed, but for its clarity. Past efforts to measure general AI capabilities have often struggled with the limitations of narrow, static benchmarks. Many traditional tests either saturate too quickly or don’t generalize well across domains.
By contrast, METR’s time horizon approach ties model performance directly to human-scale effort. It gives us an intuitive sense of how capable a model is—“Can it do a task that would take me an hour?”—and provides a single metric that allows comparisons over time.
The researchers also took care to address potential limitations. They ran supplementary tests using alternate datasets, like SWE-bench Verified (a widely used benchmark for evaluating AI software engineering skills), and even tested models on real issues from internal METR repositories. In most cases, the time horizon trend held, though tasks with more “messiness” (e.g. unclear goals, changing environments, or high-context requirements) remained more difficult for AI agents.
Still, even on these harder tasks, progress has been consistent.
What’s Driving the Gains?
According to the paper, the improvements stem from several key areas: better tool use, improved logical reasoning, greater robustness to failure, and the ability to adapt mid-task. For example, recent models were far less likely to repeat the same failed actions, and more likely to course-correct after errors—something older models struggled with.
Notably, the study found that task reliability drops sharply at higher thresholds. Claude 3.7 Sonnet, for instance, has a 50% time horizon of nearly an hour, but only a 15-minute horizon at 80% success. In other words, models can often succeed at longer tasks—but not reliably yet.
Extrapolation and Its Limits
If the trend continues, METR forecasts that models could reach a one-month time horizon—roughly 167 work hours—sometime between late 2028 and early 2031. This would mark a major threshold: an AI system capable of autonomously completing projects that take a skilled human a full month.
However, the authors are careful to include caveats. Real-world work often includes ambiguity, team coordination, high-stakes consequences, and shifting priorities—conditions that even advanced models still struggle with. Tasks used in this study were automatically scorable and structured to allow clean comparisons. That doesn’t always reflect how problems unfold in the wild.
Whether or not the trend generalizes to all types of work, METR argues, the consistency of the growth curve itself is meaningful. If it does generalize, we may be closer to transformative AI systems than many expect. If it doesn’t, the study still provides a concrete case for improving our benchmarks—and designing evaluations that more accurately reflect the complexity of real-world labor.
The Bottom Line
METR’s “time horizon” benchmark may represent a turning point in how we evaluate AI capabilities. By rooting performance in human-scale time and practical task completion, it offers both an intuitive metric and a quantitative trendline for tracking progress.
The field still faces fundamental questions—about reliability, generalization, and alignment—but this research brings us closer to answering one of the most important ones: not just what today’s AI can do in theory, but what it can actually accomplish in practice.